Optimising box sizes – letting the air out
14.11.2022 – Reference Digitec Galaxus
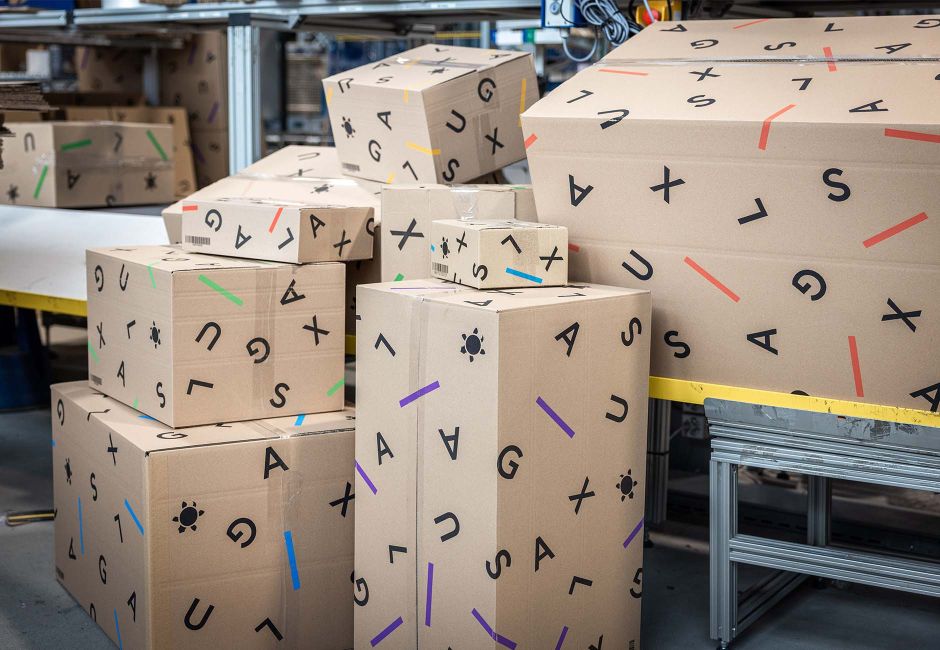
Digitec Galaxus is short of air. And that’s a good thing. The online retailer has optimised the size of its boxes. Why? Because any unused space must be filled. That isn’t environmentally friendly, it’s unpopular with customers, packing orders takes longer, and there’s more volume to transport. It took Ergon just two months to model the company’s requirements and calculate what box sizes would work better. With seven billion variables, optimising the model demanded great engineering and cloud computing expertise. The result was a 28 percent reduction in the need for filler.
Digitec Galaxus is Switzerland’s biggest online retailer. Customers love the huge range it offers. Where shipping is concerned this enormous choice also poses a challenge, however. Empty space is unavoidable when packing items for delivery. These voids must be filled in some way, such as with small air cushions. This prevents goods being damaged in transit. But it takes time, and customers don’t like getting large boxes containing excessive amounts of filler.
While certain shipments can be packaged by machine into customised boxes, others are packed manually. For practical reasons Digitec Galaxus uses a fixed set of box sizes. But might different sizes not be better to minimise empty space?
In line with the Paris Climate Agreement, Digitec Galaxus is investing in reducing its own carbon footprint. This project aimed to reduce filler to save on valuable resources and cut the waste that the end customer has to dispose of. In doing so the company is not only doing its bit for the environment, but also raising customer satisfaction.
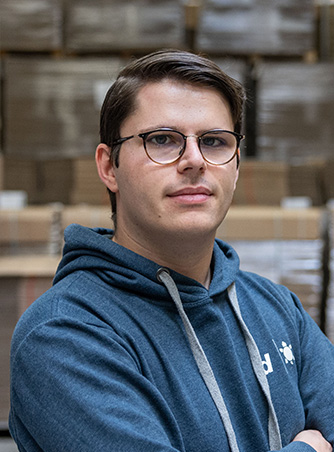
“Alongside Ergon we were able to solve a complex optimisation project in no time. This enables us to save packaging and costs, look after our staff and improve customer satisfaction.”
Modelling: what’s best for business?
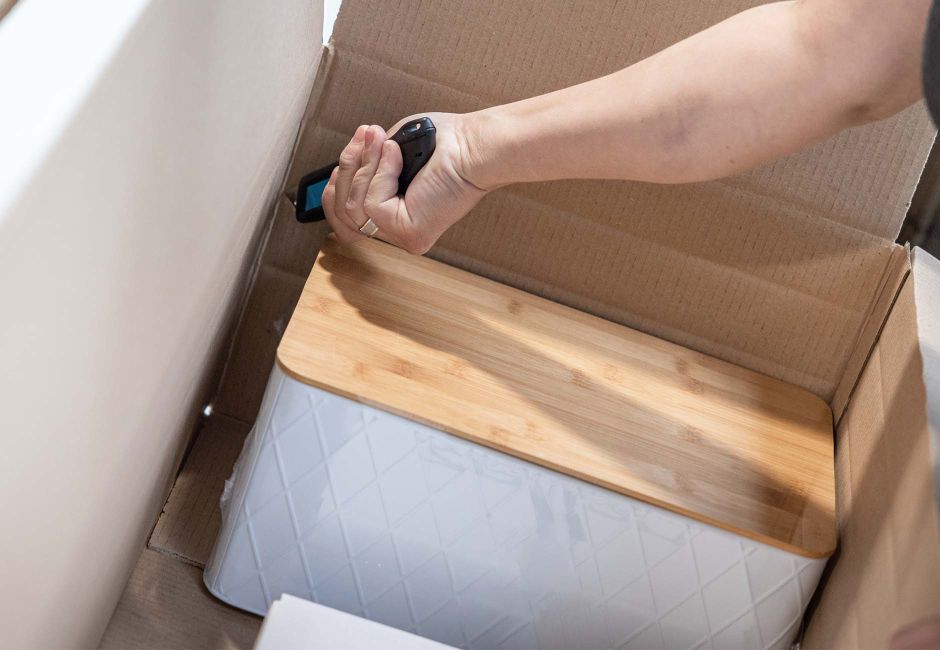
The search for perfect box sizes can be formulated as a constrained optimisation. This approach models KPIs and additional requirements using equations, while numerical methods identify the optimum of the defined mathematical model.
The primary aim here was to reduce unused space. The more box sizes can be used, the greater the saving. However, limited room at the packing stations necessarily restricts the number of box sizes. Effective modelling demands both technical and domain expertise. For example, crease lines in the cardboard quadruple the number of available sizes without taking up any more storage space. All the packer then has to do is make a few small cuts. Another factor is that the postage is cheaper for certain package sizes. Modelling all the box requirements is paramount for a solution to be optimum from a business perspective.
Mathematical models can be used for much more than optimising box sizes, however. In fact, they are an excellent way of formulating various, often contradictory requirements. Humans must first define business KPIs and other requirements, then the machine will work out the optimum.
Large-scale optimisation: finding the solution
Modelling requirements is only the first part of the work. The second is the optimisation itself, which was particularly demanding in this case.
The resulting model is a mixed integer program (MIP) with seven billion variables. MIPs are an established type of problem. If the model is small enough, it can be solved by generic software known as a solver.
In this case, however, the combination of all possible box sizes (around 70,000 to an accuracy of 1 cm) and the number of shipments considered is too large. From 100,000 shipments and upwards the data appears to be statistically representative. Fewer shipments impair the result, but more only takes more computing power.
Mixed integer programs are supported by a sound body of research, and the academic literature offers many tried-and-tested techniques. They offer the benefit of experience and involve less trial and error. Good reasons to go for established mathematical models.
Benders decomposition was key to this project. It breaks a model down into simpler sub-problems and solves them iteratively. In this case most of these sub-problems could be solved analytically and in parallel. This left a much smaller MIP that an open-source solver could solve.
Optimising box size nonetheless remained a large-scale problem. It took a 96-core server with 384 GB RAM just a few hours to work out the optimum. It is a perfect use case for cloud computing, because box sizes are typically optimised only once a year.
Win-win-win
The optimisation project paid off. The new box sizes were ordered and the results show a reduction of around 28 percent in the need for filler. This cuts waste, saves materials and processing costs, and makes for happier customers. Using less cardboard and filler also helps the environment, in the spirit of the Paris Climate Agreement, and shrinks Digitec Galaxus’s carbon footprint. It’s a win–win–win situation.
In just two months Ergon brought a comprehensive range of capabilities to bear, from mathematical modelling to large-scale optimisation and cloud computing. And all from a one-stop service, in the shortest time, and with a measurable impact.
Thinking about optimising your business? Ergon is an expert in operations research. We will advise and assist you with the entire process, from capturing your requirements and modelling to supporting you with implementation.