This article was published in the Ergon Magazine SMART insights 2019. Order your free copy now ->
No trend is currently being discussed as widely and with such indiscriminate enthusiasm as artificial intelligence (AI) but what a lot of people don’t know is that a large proportion of AI development is open and accessible to everyone. Michael Schröder and Wilhelm Kleiminger describe how companies can make an agile and scalable start to their AI journey with the right approach and data science in the cloud.
Although the AI conversation is dominated by the big players, there are plenty of opportunities for virtually every firm to deploy AI to improve and transform their business activities. Below, the authors present the two principal approaches to AI and address individual methods and success factors.
AI in practice: applied AI and custom AI
Aside from AI research, there are fundamentally two approaches through which companies may benefit from AI:
- Applied AI
This is where AI products, such as image or text recognition, are used “out of the box” to automate specific processing steps and/or to make these smart at an application level. - Custom AI
This is where companies use AI to analyse data, recognise patterns, make predictions and, ultimately, deliver customised AI solutions based on their specific business practices.
Applied AI: quick-wins with existing AI products
Public cloud providers such as Google, Microsoft and Amazon already offer out-of-the-box AI products that can easily be incorporated into existing projects. These include speech and image recognition, machine translation and modular systems for conversational interfaces, for example. The advantages of these products are obvious: they allow major improvements to be made in specific areas without requiring extensive changes to existing system infrastructure – image recognition can thus be used to optimise workflows while a chatbot can lighten the load in a service centre. This low barrier to entry is simultaneously the greatest drawback of applied AI approaches, however – they can easily be mimicked by the competition and hence do not provide a sound basis for unique competitive advantage.
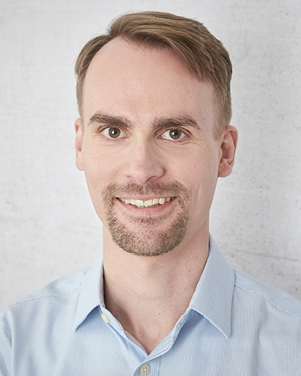
“Practically every company can benefit from AI.”
Custom AI: competitive advantage thanks to individualised approach
If companies wish to create additional commercial benefits and elicit fundamental competitive advantage from AI, relying on the standard range of products available is longer adequate. Instead, they must identify at what points in their business processes data suitable for custom AI is being generated and analyse how they can improve, or even fundamentally redefine, their competitive position using AI and any newly captured data.
Use cases for custom AI can be directly derived from a company’s business architecture and priorities: what problems within individual business processes produce sufficient data for AI-based solutions to be worthwhile? How might a company monetise existing data or leverage such information more effectively by combining it with new data? What data-based products or business models might be envisioned?
Approaches such as combining the IoT and AI for predictive maintenance, within production, or smart things, for the end user, that utilise fundamentally new concepts and technologies are of particular interest here. In combination with changed customer and/or partner relationships, such cases harbour potential for long-lasting competitive advantage or even the disruption of entire sectors.
From idea to operational AI solution
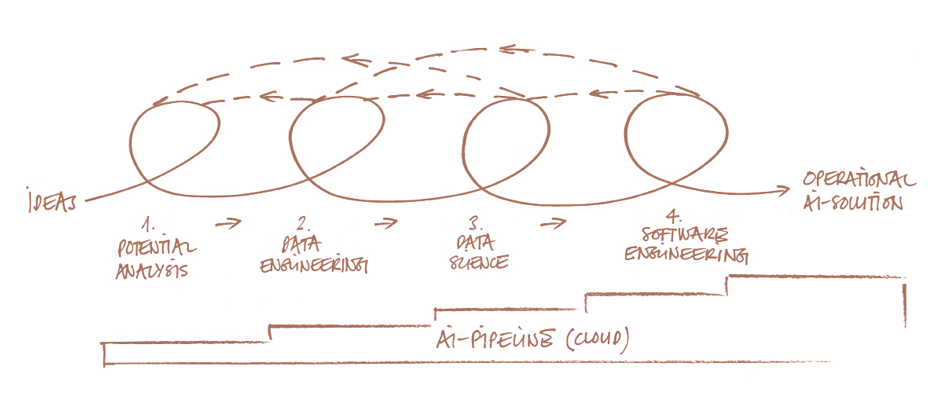
Combining structured understanding and consolidation with an iterative and agile implementation methodology, the following approach has proved its worth as a way of progressing from the identification of ideas to an up-and- running AI solution.
The concrete steps are:
- Potential analysis
The important thing at the beginning is to investigate ideas for their technical and data-related potential. Simultaneously, this is when the first, brief data analysis takes place – ideally, at this point, in the correct AI pipeline, which can be scaled later.
- Data engineering
Data is the bedrock for training AI algorithms and differences in data quality and/or data from disparate silos that cannot be combined for technical reasons are typical hurdles here. Data engineering addresses these deficits in conjunction with technical/data-related and functional/organisational obstacles. In such cases, there will often be a “loop-back” to the potential analysis.
- Data science
A central plank, data science subsumes suitable techniques and approaches for extracting specific technical information for the AI models from the data available. New potential or shortcomings in the information base may only become apparent at this stage and, here too, the process may have to go back a step or two.
- Software engineering
The final step is to transpose the successful AI algorithms from the data science stage into a scalable and robust solution. Given that there will be no shortage of productive data, further fine-tuning and loop-backs to earlier stages may take place. Here is where it pays off for the company to have chosen a suitable, scalable AI pipeline from the get-go, so it can be highly agile in hopping back and forth between the individual stages.
The journey can begin
Practically every company can benefit from applying AI to its business activities. Although applied AI can be brought online speedily and efficiently, while promising only narrowly focused benefits, truly transformative value can be generated with custom AI. Firms with a claim to market leadership in their sector, in particular, can cement and enhance their position with custom AI solutions.
When it comes to choosing a path, companies should opt for an approach that enables speedy and costeffective potential analysis as well as direct and systematic data engineering and data science – potentially to be followed by the professional and secure implementation of a productive AI solution. With the “data science in the cloud” approaches now available, there are generally accessible, low-threshold and yet sustainable entry points for a successful start to a custom AI journey.
Custom AI: Data and software engineering – getting it right from the start
This article was written by Michael Schröder, Head of Consulting at Ergon, and Wilhelm Kleiminger, Principal Data Scientist at Ergon.
Interested in more?
Digitisation projects
Change makers
Tech trends
Order now
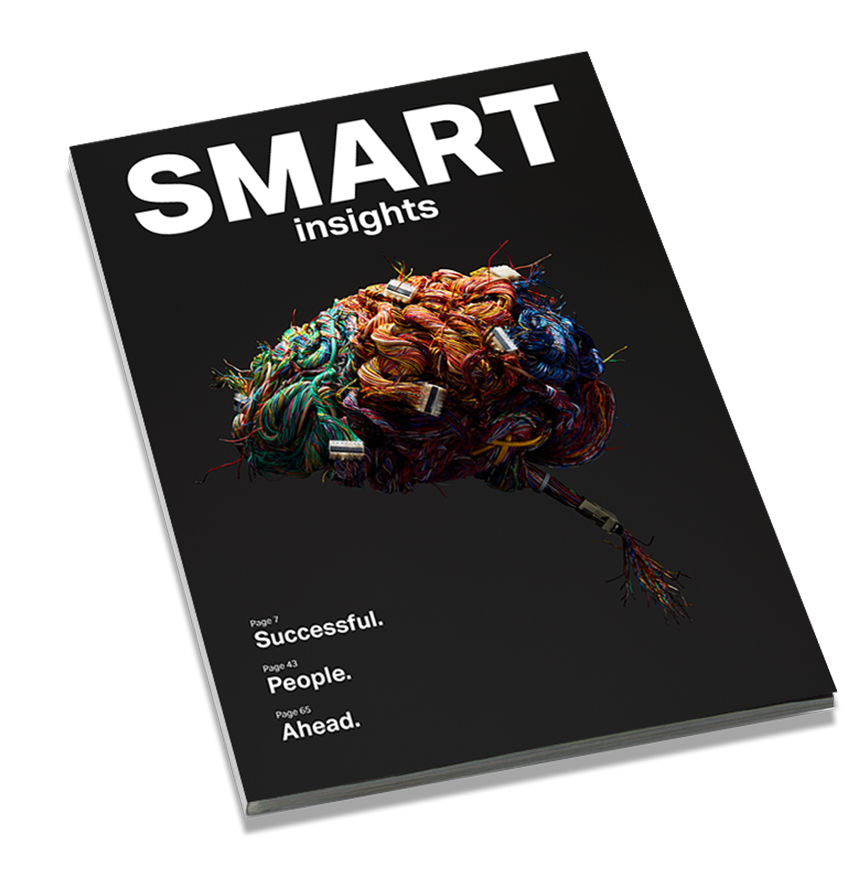