Fraud Detection 4.0
16.12.2016 – Adrian Berger
Expert article for Computerworld dated 15 December 2016
Online fraudsters are getting smarter and smarter – so security measures need to be smarter too. This was the thinking behind a machine learning development project by Ergon, which is now beginning to bear fruit.
Payment processes are a hacker’s favourite target. Not surprising, given their status as one of the most vital processes in the entire financial sector. Hackers are able to infiltrate right at the source, manipulating the process to their own ends. Preventing such abuses is therefore amongst the most critical security measures in online banking. After all, it only takes one incident that causes customers to lose faith in an institute to cause catastrophic damage.
Security measures: hurdles and rising costs
Various approaches can be taken to detect and prevent fraudulent activity. For example, one relatively straightforward measure from a technical perspective is requiring online banking users to perform an additional authentication step for each transaction after login. Essentially, this means the user has to provide additional verification of their transaction using a token after having already completed a robust authentication process. Though effective, for the user this step represents yet another hurdle to negotiate and is therefore not particularly customer-friendly.
An alternative approach involves having trained personnel manually going through all processed transactions and calling customers for confirmation over the phone if a suspicious transaction is detected. This measure is relatively expensive for the provider, for one due to the staffing cost, but also due to the need for a framework setting out what exactly it is that makes a transaction suspicious and subject to authorisation.
The most widespread approach currently used by banks is a semi-automated rule-based system which evaluates whether payments require special authorisation (e.g., a new payee, very high transaction volumes, an unusual recipient country). With these rules in place, every single step of the transaction authorisation process is transparent. The disadvantage, however, lies in the fact that the system is static and new kinds of attacks mean that changes to payment procedures and new authentication rules are required. Only the few employees who are responsible for maintaining the complex rules framework have the complete picture. Thus, the potential for errors and false positives rises, meaning an excessive number of transactions are incorrectly flagged as suspicious. This results in increased interaction rates with end customers through the hotline, in turn resulting in increased costs.
React quickly to new threats
Faced with new kinds of attacks and ever more professional and organised fraudsters, it is already apparent that semi-automated systems are no longer sufficient for effective protection. This was the motivation for Ergon’s security department to develop and test a new procedure: an intelligent fraud detection system that makes use of machine learning. The concept was to create a self-learning system that can handle large volumes of data faster and better than human experts while reliably tracking errors and attempted attacks. It needed to be able to analyse large volumes of anonymised data by identifying and generalising patterns and norms, and to accurately evaluate a scenario and learn from it, without the need for new rules being manually defined or any other form of interaction.
Results are in from first pilot test
So, what was required to carry out this project? To start with, we needed a large volume of transaction data, expertise in the field of data science, outstanding insider knowledge of the financial sector and a customer who was prepared to join us in our experiment and provide highly sensitive data. It did not take long for all of the pieces to be put into place, and so the research project was ready to begin.
The first step was to develop a data mining-based system that, using a subset of 10,000 transactions as its learning data, could learn patterns and normal behaviour and subsequently detect attempted fraud. The next stage involved testing how well the fraud detection system was learning: 300 further transactions were simultaneously analysed by human experts using existing methods and the newly developed system. And the results were impressive: even in this first test, the self-learning system delivered more accurate fraud detection results than the group of human experts.
Now the emphasis is on further improving the concept so that it can deliver even more accurate results. As is to be expected with machine learning, this requires more data from other systems: the more learning data is fed into the system, the more intelligent it will become. The research work for this is already well under way, and links have already been established with other transaction systems, with a view to obtaining more data and being able to react dynamically to events.
The solution’s purpose is to make transactions more user-friendly: machine learning and intelligent, interconnected systems could render up to 90% of today’s demonstrably superfluous transaction authorisation checks obsolete.
In order to achieve the most comprehensive fraud protection possible, machine learning should be implemented alongside other measures. In the future, Ergon will be offering the fraud detection solution in combination with Airlock Suite, thereby enabling prevention and detection mechanisms to work in tandem. This holistic approach to fraud detection can be implemented in payment transactions with an exceptionally low false positive rate, meaning costs have been reduced for fraud detection and less manual interaction is required.
Fraud detection in combination with Airlock Suite
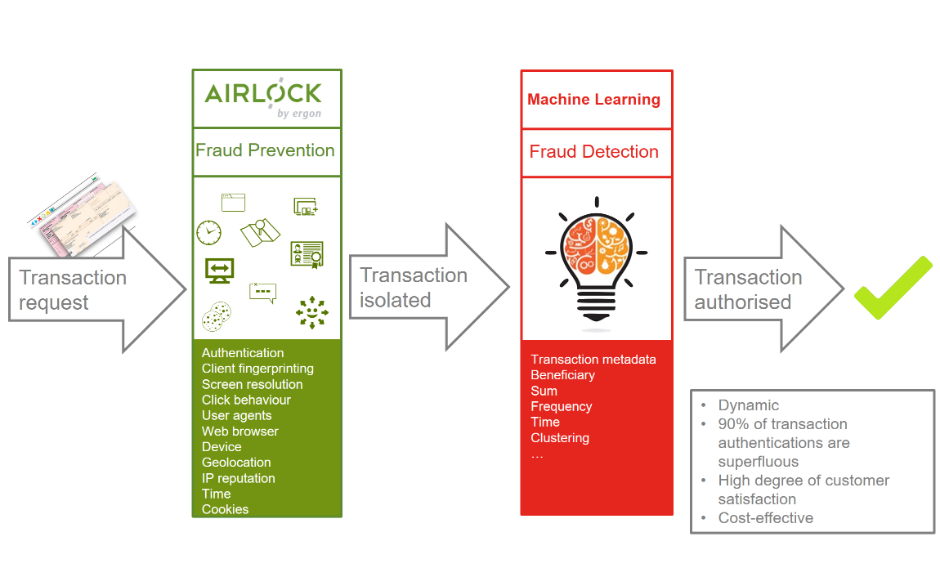
Airlock Suite is an upstream security system comprising a combined solution of consisting of a web application firewall and identity and access management. It is Switzerland’s most used security solution for application security and is used by over 350 banks, insurance companies and other organisations worldwide.
Prevention measures (Airlock Suite)
The web application firewall allows parameters of the runtime environment to be logged and verified, including browsers, click behaviour, malware detection and screen resolution. Using client and session fingerprinting, all runtime environment parameters are verified in order to detect manipulation attempts during sessions.
WAF also offers Dynamic Value Endorsement (DyVE) – a kind of dynamic whitelisting – as a further fraud-prevention measure. Take online banking transactions as a simple example: using DyVE, the user can instruct Airlock WAF that requested transactions can only be charged to accounts that have previously been selected by the banking server.
Risk-based authentication is yet another means of fraud prevention within Airlock Suite: robust, two-factor authentication is now the norm for business-critical web applications such as online banking. In day-to-day practice, however, users often consider this to be a somewhat painstaking security measure. That is where risk-based authentication – or adaptive authentication – comes in. The context of an access attempt is closely analysed and compared with the same user’s recent sessions. If Airlock IAM concludes, for example, that the user is trying to log on to the intranet from their regular workstation or their home office, then it is fine to forgo the two factor authentication. By analysing the authentication history, parameters such as geolocation, IP reputation, time, cookies or browser can be checked for consistency with the user profile’s normal behaviour.
Detection measures (machine learning)
Airlock Suite also protects the downstream side, with fraud detection that uses machine learning offering protection within the application in the event that a fraudster has been able to penetrate the system despite the other security measures. Here, recipients, transaction metadata, volumes, frequency and other parameters can all be checked in order to reliably and automatically detect attempted fraud. Machine learning and the Airlock Suite are a perfect match as machine learning is specifically focused on fraud detection, while the Airlock Suite is designed for application security.
Adrian Berger holds a Diploma in Computer Science from ETH (Zurich) and is Managing Director Finance Solutions at Ergon Informatik AG.